Generative AI (GenAI) has made significant strides in recent years, revolutionizing various fields such as natural language processing, image generation, and artistic creation. However, one area where GenAI often falls short is accurately adding colors to black-and-white images. This task, which may seem straightforward at first glance, is fraught with challenges that reveal the limitations of current AI technologies.
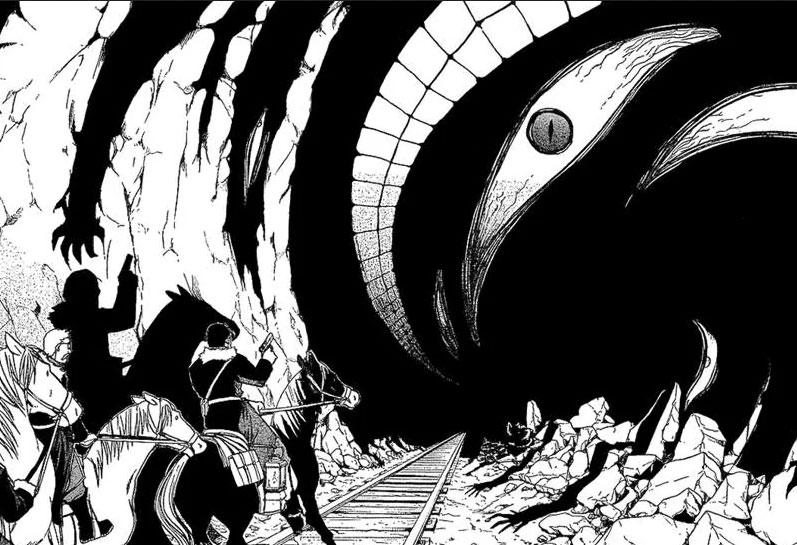
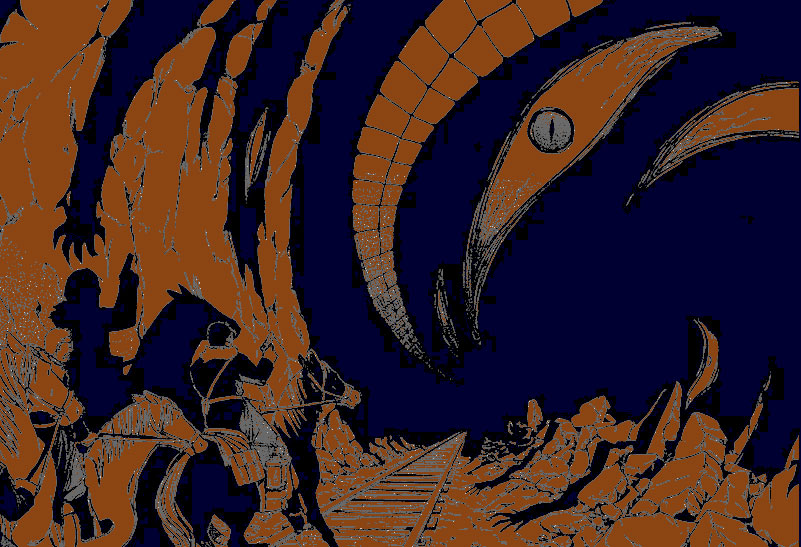
Image Source: /r/FullmetalAlchemist | Colored: GPT-4
The Complexity of Colorization
Colorization of black-and-white images is more than just filling in colors; it requires understanding the context, the objects in the picture, and, often, the historical or cultural significance of the elements present. For instance, the AI must distinguish between a blue sky and a blue shirt or a green tree and green grass and color them accordingly. This complexity demands a contextual understanding that current AI models often lack.
Lack of Contextual Knowledge
One of the primary reasons GenAI struggles with colorizing black-and-white images is the lack of contextual knowledge. AI models are trained on vast datasets of images, but they do not possess true understanding. They rely on patterns and correlations found in the training data, which can lead to inaccurate color choices. For example, an AI might colorize an object based on its most common color in the dataset, which may not be correct for the specific context of the image being processed.
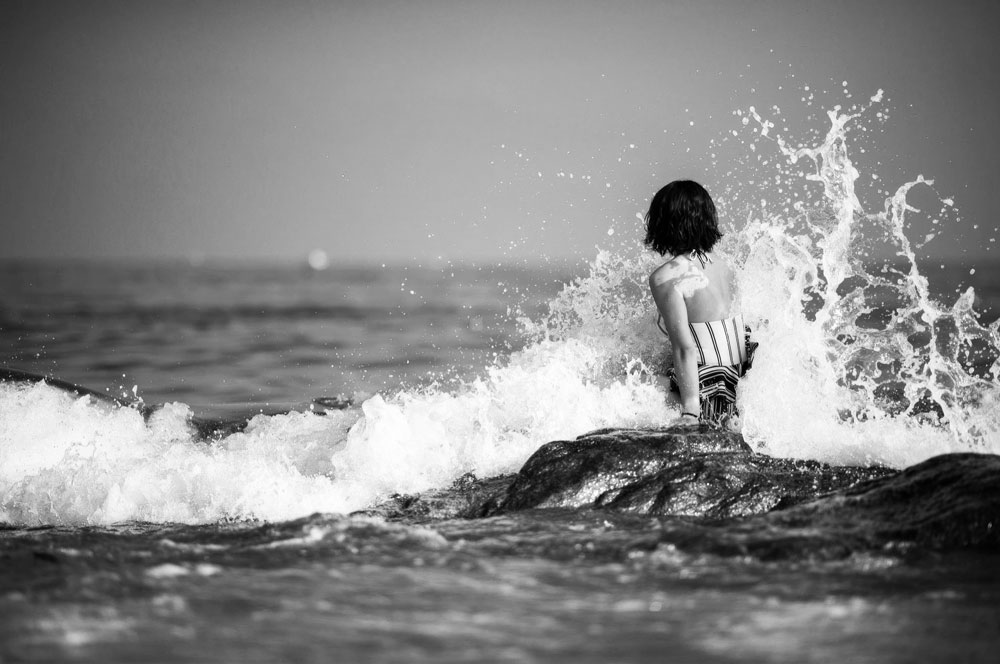
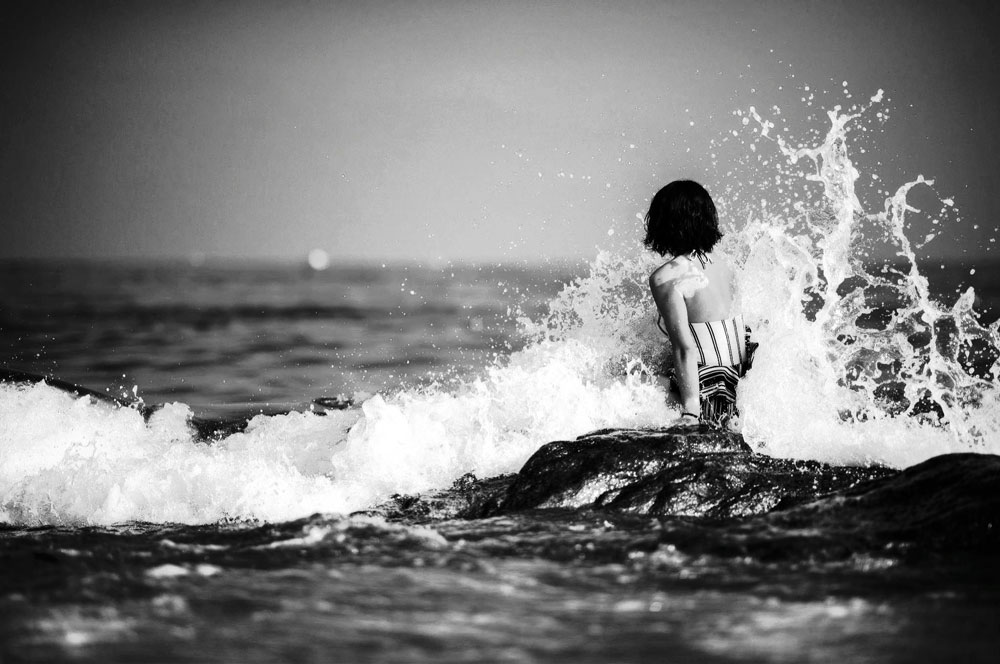
Image Source: /r/blackandwhite | Colored: GPT-4
Technical Constraints
Colorizing black-and-white images requires advanced techniques combining computer vision and machine learning. The process involves predicting the colors for each pixel while ensuring that the colors are coherent and realistic. This is computationally intensive and prone to errors, especially in detailed or complex images.
Some of the research papers discussing this problem
- Colorful Image Colorization
- Generative AI for visualization: State of the art and future directions
- Image Colorization Using Generative Adversarial Networks
Future Directions
Despite these challenges, ongoing research in AI and machine learning is gradually improving the capabilities of colorization models. Techniques such as deep learning, GANs (Generative Adversarial Networks), and reinforcement learning are being explored to enhance the accuracy and realism of AI-generated colorizations. Additionally, incorporating more contextual understanding and leveraging user feedback could help bridge the gap between AI-generated and human-created colorizations.
Conclusion
While GenAI has shown remarkable potential in various creative tasks, colorizing black-and-white images remains a challenging frontier. The lack of contextual knowledge, ambiguity in color choices, limitations of training data, and technical constraints all contribute to the current shortcomings. However, as research progresses, we can expect more sophisticated models to understand better and replicate human vision and artistic expression’s complexities.