Artificial intelligence continues to evolve rapidly, with two groundbreaking papers making waves: OpenAI’s GPT-4 and the Gemini model. These papers highlight significant advancements and distinct approaches in AI research.
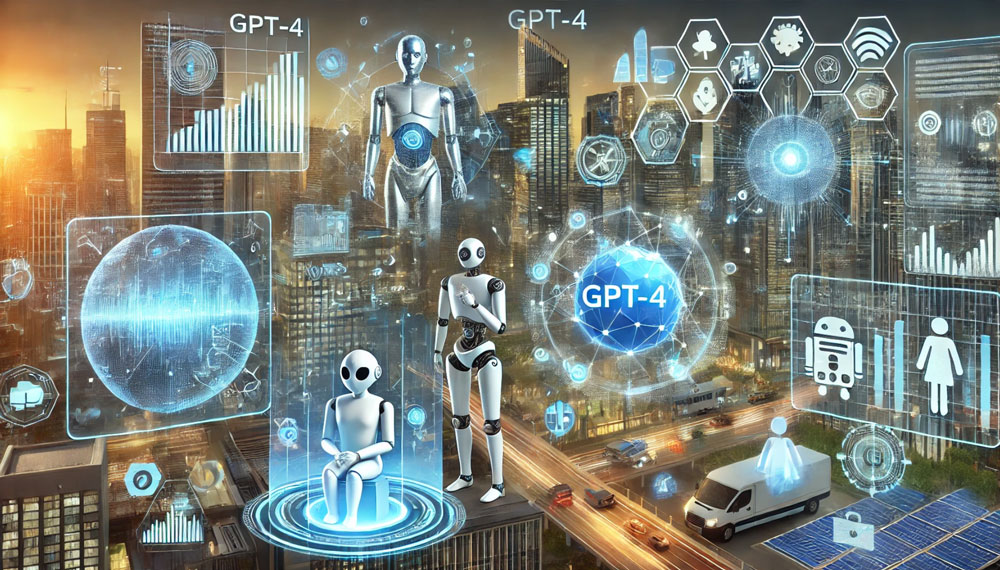
Image generated via GPT-4
The Versatility of GPT-4
GPT-4 stands out with its ability to process text and images, a feature that broadens its application across various fields, from education to professional exams. A key highlight of GPT-4 is its use of Reinforcement Learning from Human Feedback (RLHF), a method designed to enhance the model’s safety and alignment, thus reducing the risk of generating harmful outputs. Trained extensively on Azure AI infrastructure, GPT-4 has demonstrated human-like performance on several standardized tests.
Gemini’s Focus on Efficiency and Interpretability
In contrast, the Gemini model emphasizes efficiency and interpretability. While details on its specific architectural innovations remain less highlighted, its focus suggests potential advancements that could set it apart from other models. Gemini’s training methodologies and benchmark evaluations also point towards a refined approach to model performance and application.
Similarities in Research
The GPT-4 and Gemini models utilize large-scale pretraining on extensive datasets, employ transformer-based architectures, and incorporate reinforcement learning techniques to improve performance. They support multimodal inputs and are evaluated on standardized tests and diverse benchmarks to measure their performance across various tasks. Both models aim to achieve human-like performance on professional exams and academic challenges, demonstrating their advanced language understanding and reasoning capabilities.
- Objective: Both GPT-4 and Gemini papers aim to advance the capabilities of large language models (LLMs).
- Training Methods: Both utilize large-scale datasets and focus on improving model performance through extensive training.
- Evaluation: Both models are evaluated on various benchmarks to assess their performance across different tasks.
Differences in Research
GPT-4 integrates text and image processing within a single model, emphasizing safety and alignment via Reinforcement Learning from Human Feedback (RLHF). It uses extensive computational resources on Azure AI infrastructure. In contrast, Gemini might adopt distinct architectural innovations or additional modalities, possibly prioritizing efficiency or interpretability over safety. Benchmark methodologies differ slightly, with GPT-4’s focus on professional exams and real-world applications, while Gemini could introduce new benchmarks specific to its innovations. Training data, preprocessing steps, and computational infrastructure also vary between the models.
- Model Architecture: GPT-4 primarily focuses on a single model capable of text and image input, while Gemini might include distinct architectural innovations or additional modalities.
- Focus Areas: GPT-4 emphasizes safety and alignment through techniques like Reinforcement Learning from Human Feedback (RLHF), whereas Gemini may prioritize different aspects such as efficiency or interpretability.
Conclusion
The GPT-4 and Gemini papers underscore the dynamic and diverse approaches in AI research. GPT-4’s emphasis on multimodal capabilities and safety contrasts with Gemini’s focus on efficiency and potential architectural innovations. These models represent significant strides in developing advanced, capable, and safe AI systems.
For further details, explore the GPT-4 Technical Report and the Gemini Paper.